Scaling AI unlocks new capabilities, making transformative value a rational bet.
Sequoia’s David Cahn recently published his second piece on what he calls “AI’s $600B Question”, arguing that the AI infrastructure investment frenzy has created a massive gap between expected and actual revenues: “Consider how much value you get from Netflix for $15.49/month or Spotify for $11.99. Long term, AI companies will need to deliver significant value for consumers to continue opening their wallets“. While these industry titans keep us entertained, compared to AGI proper, I believe their impact will look like child's play. Sam Altman likes to say that when OpenAI got started, he was often laughed at for talking about AGI. Now, he flashes a grin when pointing out that it doesn’t happen as much anymore. Once Altman leaves the room, however, it seems many still struggle to take AGI seriously. Here’s why I think they should.
Consider the difference in capability between OpenAI’s GPT-2 and GPT-4. No doubt GPT-2 was an impressive feat when it was released in 2019. It could translate text, generate summaries, and perform many of the tasks we have now come to expect from large language models at a basic level. However, its limitations restricted its commercial appeal. A stark contrast to the $3.4 billion per year OpenAI already generates today. Although not a perfect predictor, let's assume for a moment that AI capabilities and downstream revenue have a roughly proportional relationship. By 2030, training runs of up to 2e29 FLOP are likely feasible. In other words, by the end of the decade, it will be very likely possible to train models that exceed GPT-4 in scale to the same degree that GPT-4 exceeds GPT-2. If pursued, we might see advances in AI as drastic as the difference between the rudimentary text generation of GPT-2 and the striking problem-solving abilities of GPT-4. Taking only OpenAI’s earnings to approximate total AI revenue, and assuming revenues scale with capability, we would arrive at 34 trillion dollars in annual AI revenue in 2030 — roughly ⅓ of today’s annual global GDP. That sounds absurd! Yet this is what taking AGI seriously might sound like.
“Extraordinary claims require extraordinary evidence” — Carl Sagan. My claim is not that the next AI leap is a foregone conclusion. Achieving this scale means overcoming challenges primarily from power supply limitations and chip manufacturing capacity, which must be addressed to sustain the current growth trajectory in AI scaling. Instead, I think investors and entrepreneurs should treat it as a plausible scenario. One that deserves being taken seriously, and which they should be willing to place some of their bets on. After all, venture capitalists are in the business of making bets. As Paul Graham told me during our seed fundraise: “You don’t have to convince investors that your company will be a success, you just have to convince them that your company is a good bet”. Given the enormous upside - betting on AGI is rational. Here is just one datapoint to substantiate this hypothesis: In 2022, 356 credible AI experts were asked when they believe there is a 50% chance that human-level AI exists. Where human-level AI was defined as unaided machines being able to accomplish every task better and more cheaply than human workers. Over half of the experts believed this will happen before the year 2061. Imagine the ramifications on the world economy that cheap machines with superior capability than humans would have. You might say that 2061 is a long way from today. Is it though? I plan to be alive in 2061, and I imagine many of the startups being created today are too. Additionally, this survey was taken in 2022, and my expectation is that many AI experts would have shortened their timelines significantly after the release of ChatGPT.
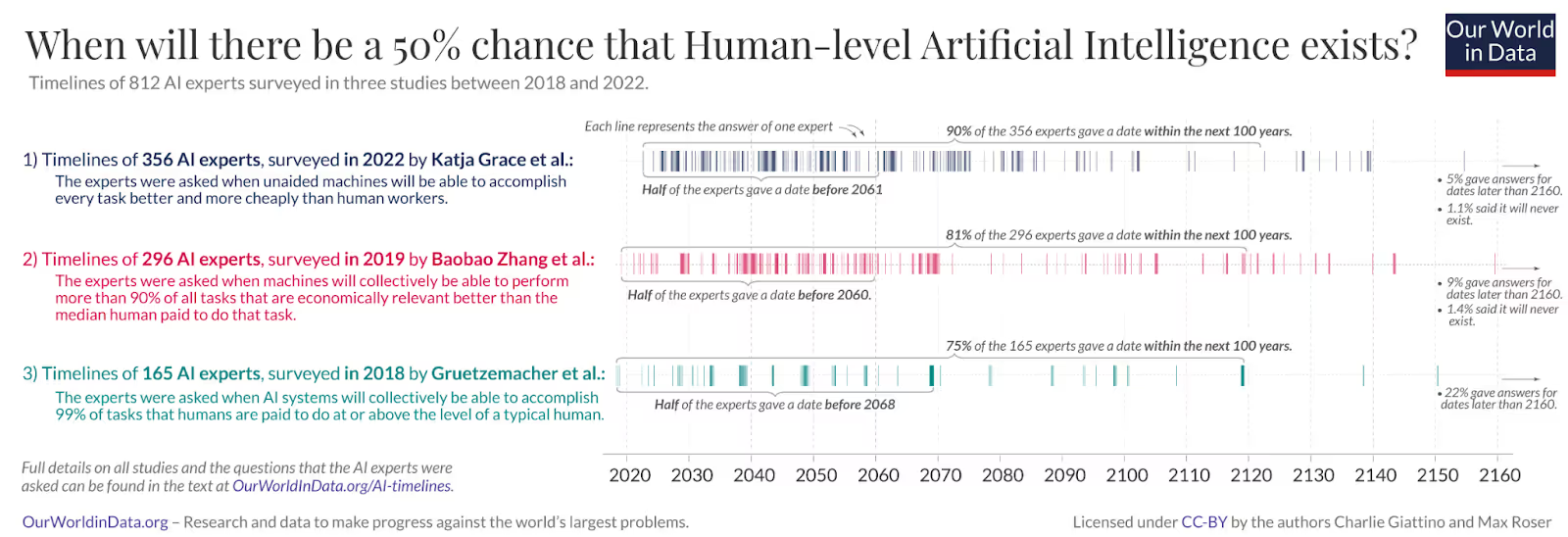
At Atla, we get to work with developers, engineers, and founders who are building AI applications every day. In particular, our team spends a lot of time assessing AI capabilities in different domains, and looking at the mistakes AIs make. We are likely among the most informed people in the world when it comes to the shortcomings of current language models. Seeing early AI systems make mistakes every day has not perturbed us from taking human ingenuity and progress in AI seriously.